The rise of generative AI has been powered by Nvidia and its advanced GPUs. As demand far outstrips supply, the H100 has become highly sought after and extremely expensive, making Nvidia a trillion-dollar company for the first time.
It’s also prompting customers, like Microsoft, Meta, OpenAI, Amazon, and Google to start working on their own AI processors. Meanwhile, Nvidia and other chip makers like AMD and Intel are now locked in an arms race to release newer, more efficient, and more powerful AI chips.
As demand for generative AI services continues to grow, it’s evident that chips will be the next big battleground for AI supremacy.
While that doesn’t quite tell us how well AMD’s competing with Nvidia in the AI gold rush, AMD CEO Lisa Su says she’s not sitting back: “The demand for compute is so high that we are seeing an acceleration of the roadmap generations here.”
She confirmed Zen 5 CPUs are still on track for this year, with server chips in second half. Acer, Asus, HP, Lenovo, MSI, and “other large PC OEMs” will begin putting Ryzen 8000 notebooks on sale in February.
Nvidia powers most of the AI projects from Microsoft, OpenAI, Amazon, and Meta, but they’re also trying to lessen their dependence on its limited supply. The New York Times explains they want to make switching between Nvidia chips and others (including their own) “as simple” as possible.
As The Verge reported, OpenAI CEO Sam Altman is interested in building chips. Microsoft’s AI-focused chip Maia 100 is expected to arrive this year, and Amazon announced the latest version of its Trainium chip.
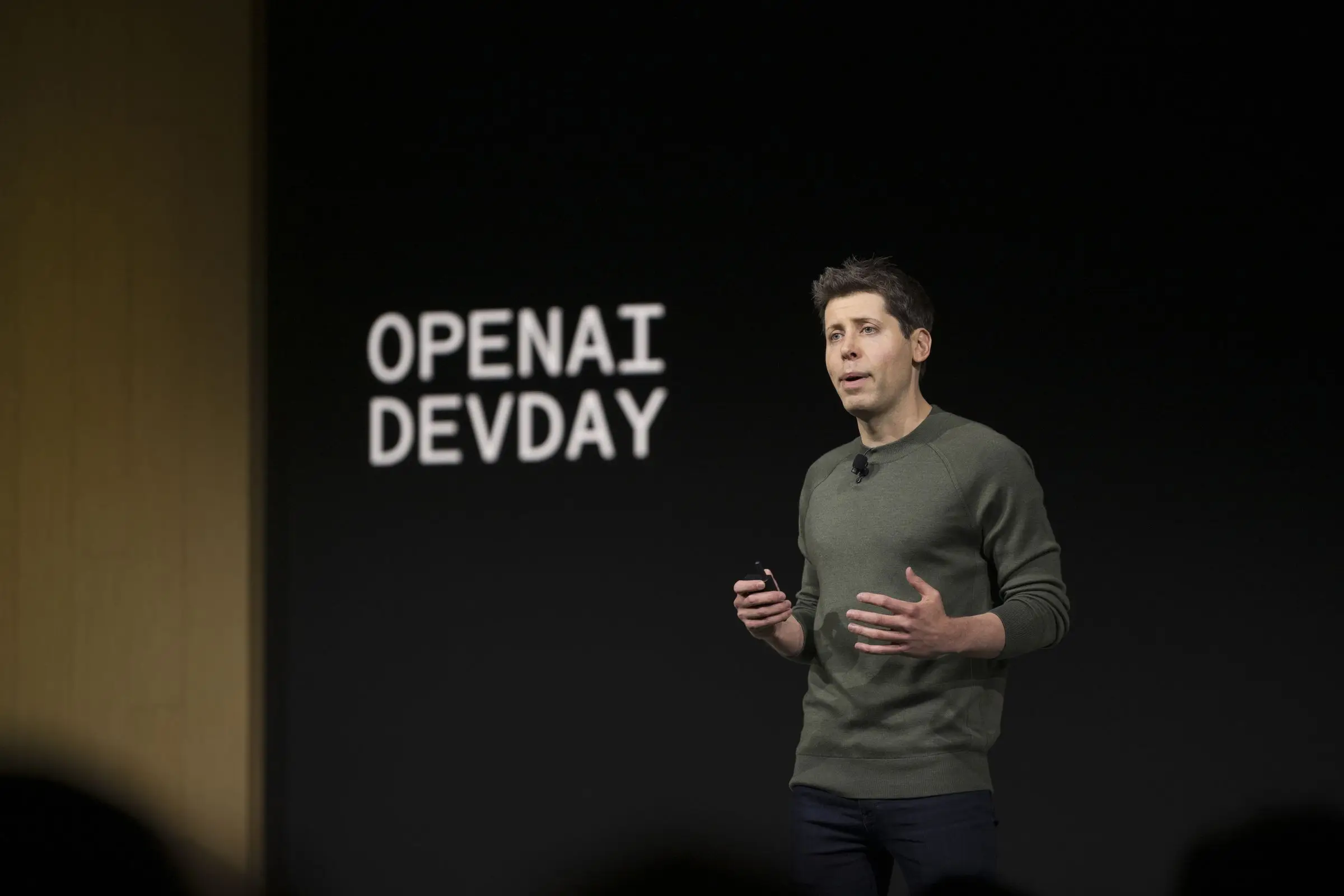
A new report from Bloomberg says that once-again CEO of OpenAI Sam Altman’s efforts to raise billions for an AI chip venture are aimed at using that cash to develop a “network of factories” for fabrication that would stretch around the globe and involve working with unnamed “top chip manufacturers.”
A major cost and limitation for running AI models is having enough chips to handle the computations behind bots like ChatGPT or DALL-E that answer prompts and generate images. Nvidia’s value rose above $1 trillion for the first time last year, partly due to a virtual monopoly it has as GPT-4, Gemini, Llama 2, and other models depend heavily on its popular H100 GPUs.
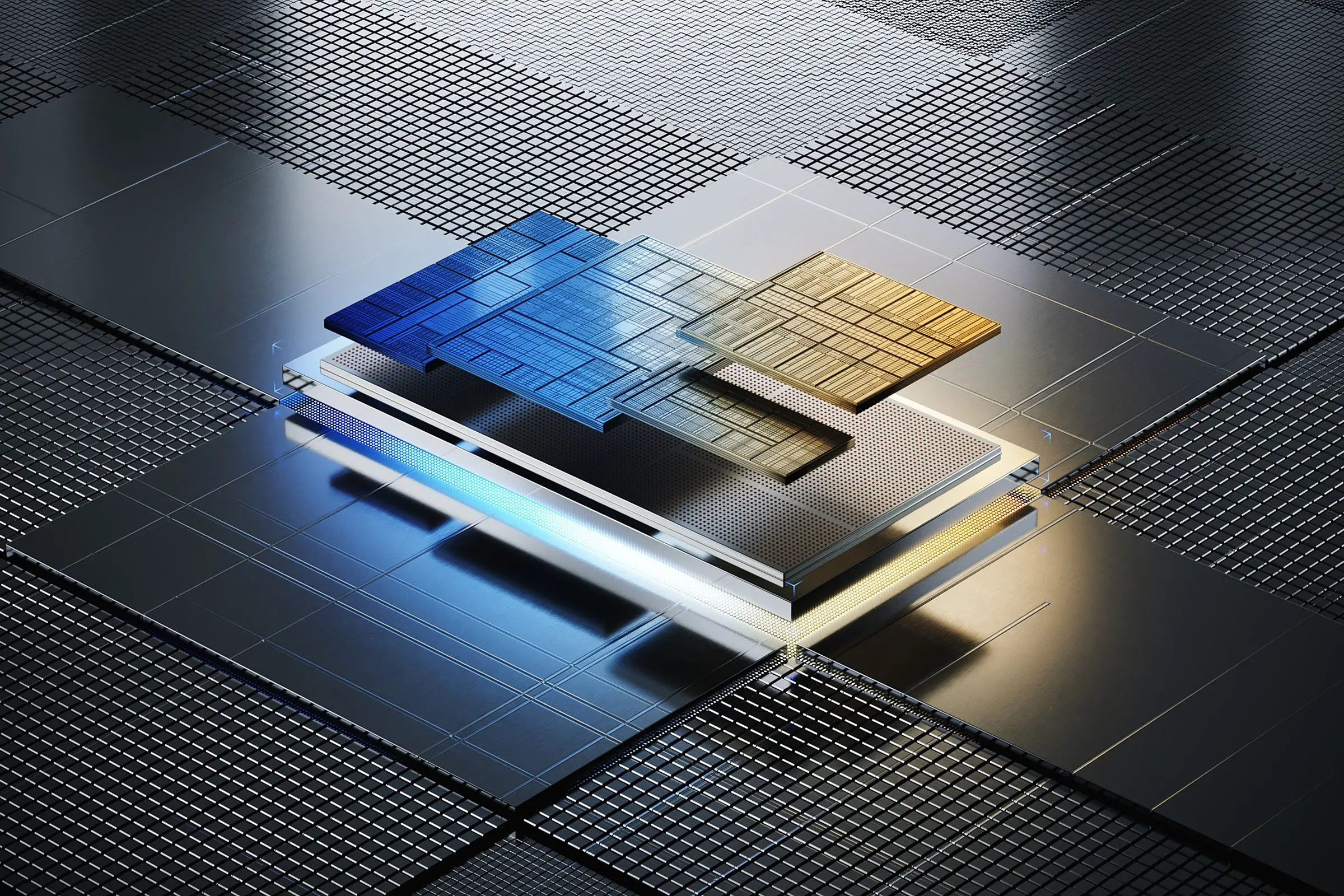
Intel is taking the wraps off its next generation of CPUs. During its AI Everywhere event on Thursday, Intel revealed all the details on the Core Ultra — no longer Core “i” — mobile processors that will be part of its Meteor Lake lineup, promising better power efficiency and performance thanks to a new setup that splits tasks across different chiplets.
Intel says its Core Ultra 7 165H chip offers an 11 percent improvement in multi-threading performance when compared to competing laptop processors, like the AMD Ryzen 7 7840U, Qualcomm Snapdragon 8cx Gen 3, and Apple’s in-house M3 chip. It also offers a 25 percent reduction in power consumption when compared to the previous Intel Core i7-1370P and has up to 79 percent lower power than AMD’s Ryzen 7 7840U “at the same 28W envelope for ultrathin notebooks.”
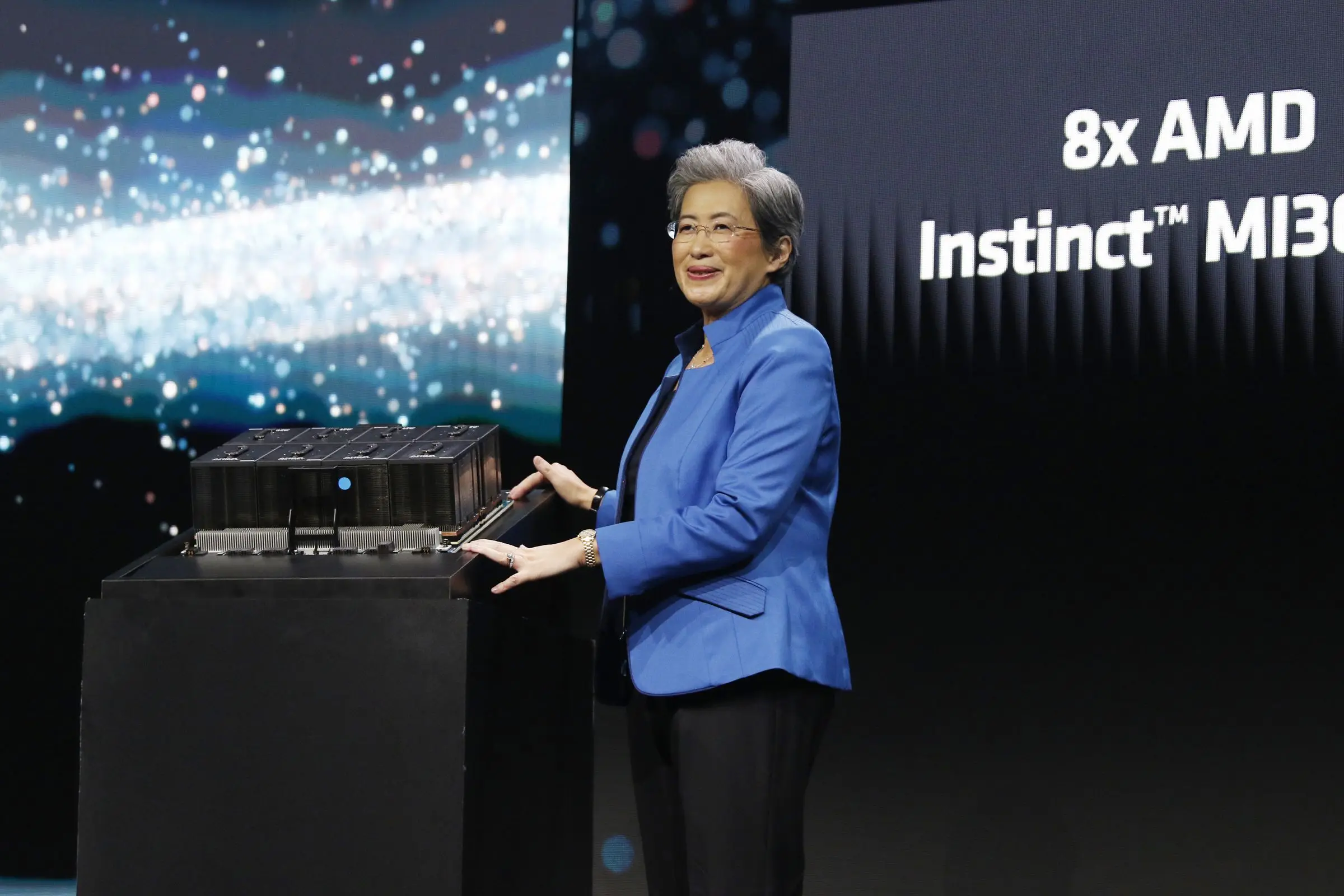
AMD wants people to remember that Nvidia’s not the only company selling AI chips. It’s announced the availability of new accelerators and processors geared toward running large language models, or LLMs.
The chipmaker unveiled the Instinct MI300X accelerator and the Instinct M1300A accelerated processing unit (APU), which the company said works to train and run LLMs. The company said the MI300X has 1.5 times more memory capacity than the previous M1250X version. Both new products have better memory capacity and are more energy-efficient than their predecessors, said AMD.
This chart from Omdia Research estimating Nvidia’s largest customers this year has been making the rounds in my social media feeds.
As I wrote in an earlier issue of Command Line, these H100s are essentially the tech industry’s new gold, since they are the preferred workhorse for powering generative AI. The gap in shipment volume between Meta, Microsoft and everyone else is quite something, and tracks with what I’ve heard from sources in recent months.
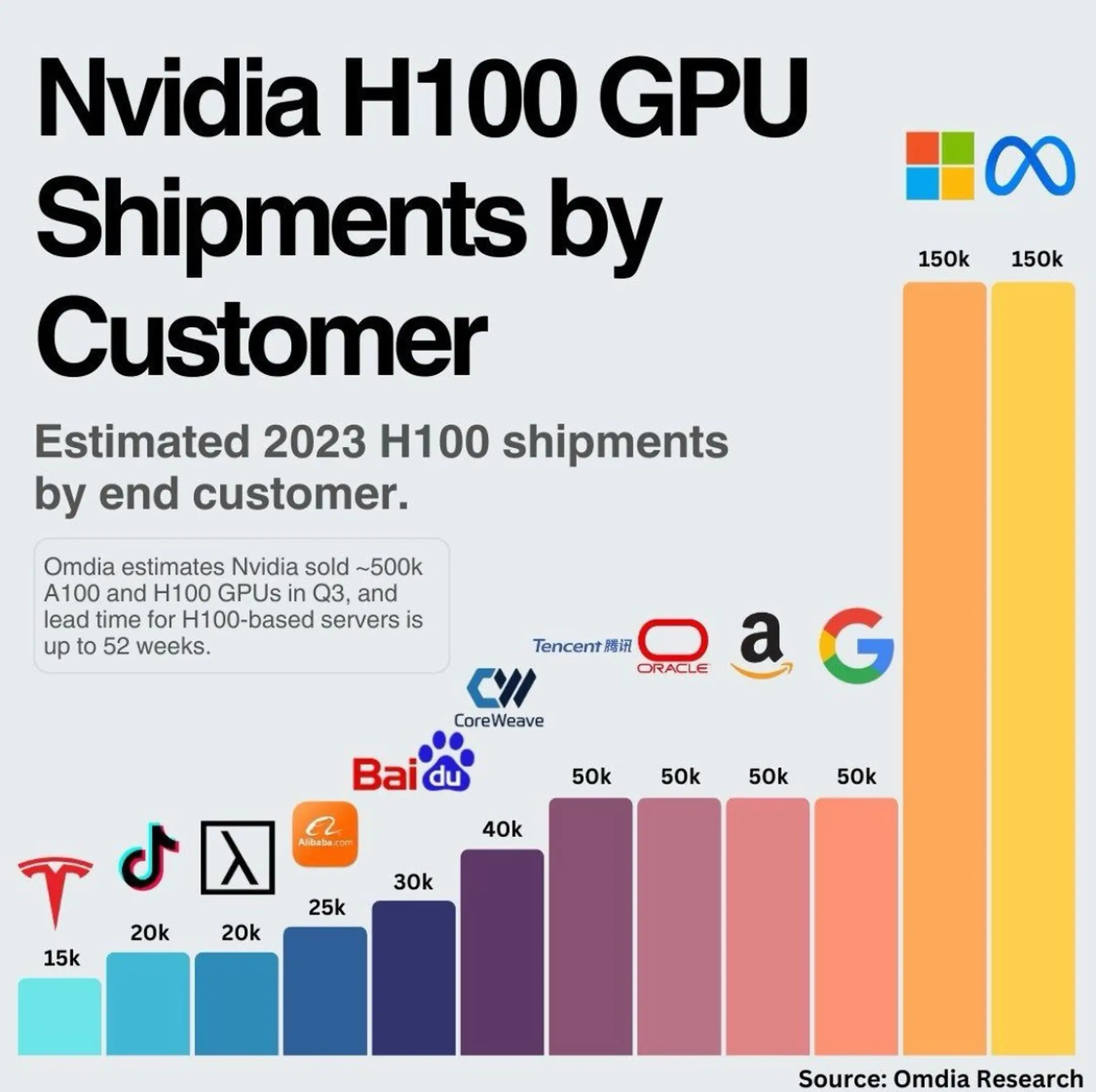
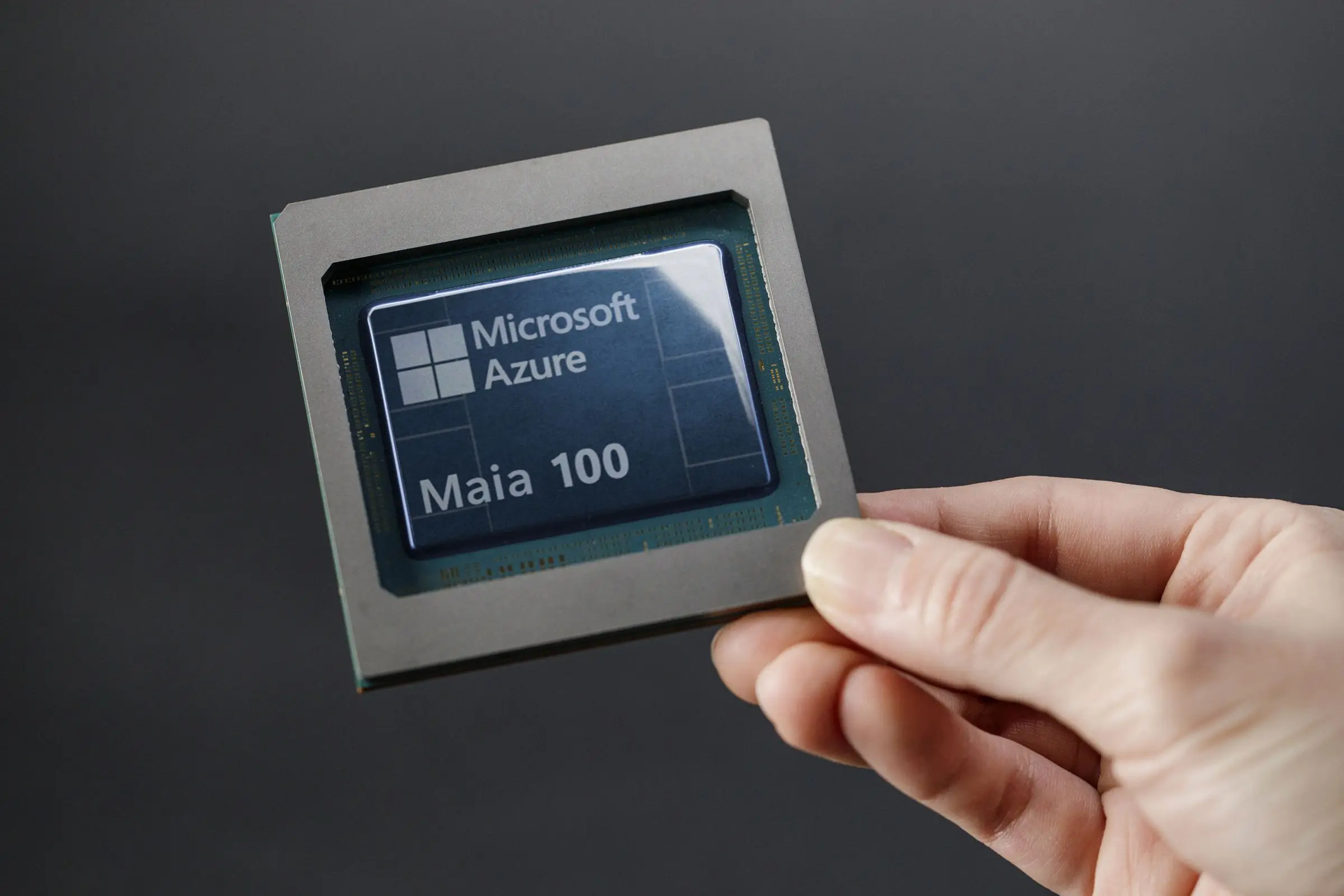
The rumors are true: Microsoft has built its own custom AI chip that can be used to train large language models and potentially avoid a costly reliance on Nvidia. Microsoft has also built its own Arm-based CPU for cloud workloads. Both custom silicon chips are designed to power its Azure data centers and ready the company and its enterprise customers for a future full of AI.
Microsoft’s Azure Maia AI chip and Arm-powered Azure Cobalt CPU are arriving in 2024, on the back of a surge in demand this year for Nvidia’s H100 GPUs that are widely used to train and operate generative image tools and large language models. There’s such high demand for these GPUs that some have even fetched more than $40,000 on eBay.
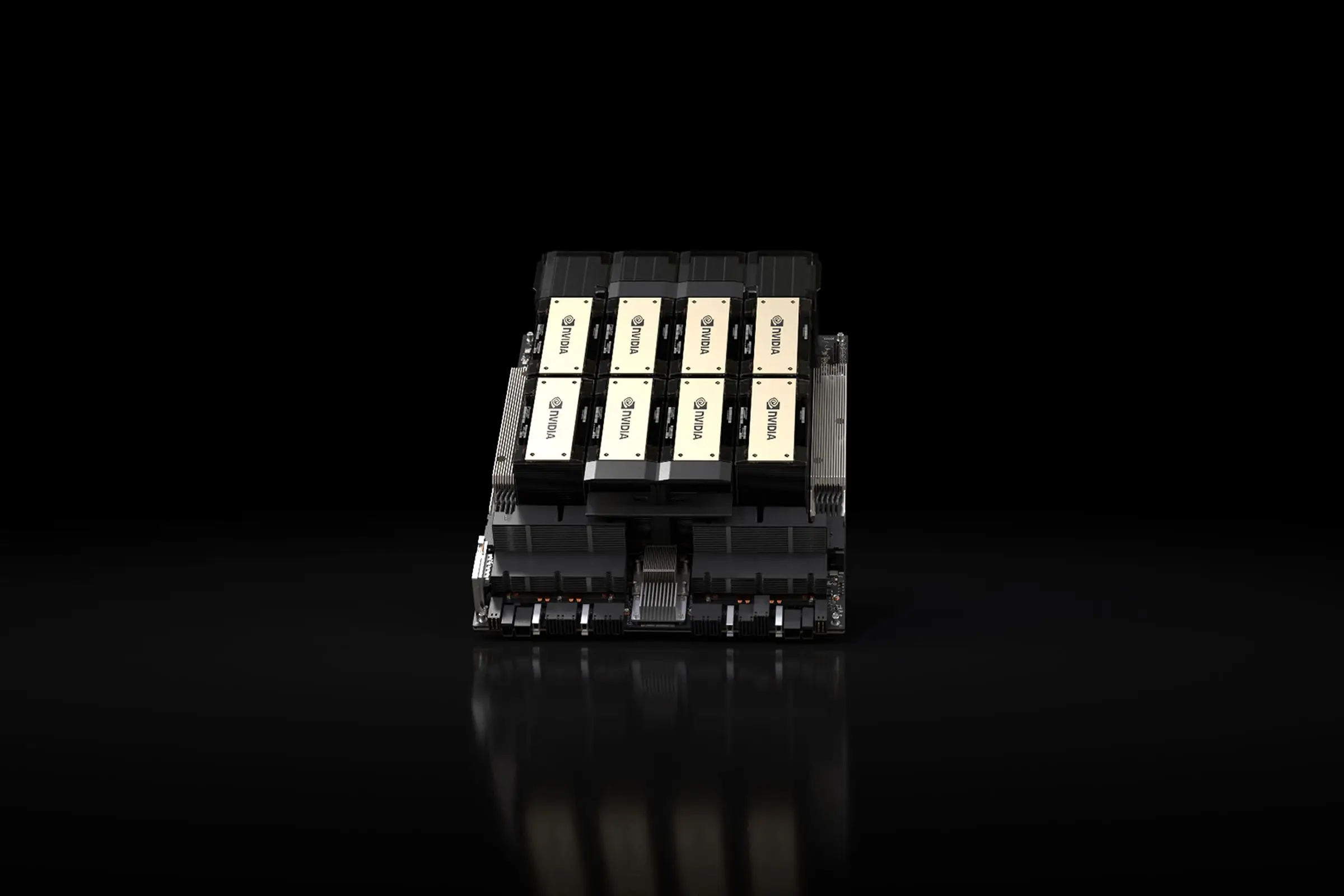
Nvidia is introducing a new top-of-the-line chip for AI work, the HGX H200. The new GPU upgrades the wildly in demand H100 with 1.4x more memory bandwidth and 1.8x more memory capacity, improving its ability to handle intensive generative AI work.
The big question is whether companies will be able to get their hands on the new chips or whether they’ll be as supply constrained as the H100 — and Nvidia doesn’t quite have an answer for that. The first H200 chips will be released in the second quarter of 2024, and Nvidia says it’s working with “global system manufacturers and cloud service providers” to make them available. Nvidia spokesperson Kristin Uchiyama declined to comment on production numbers.
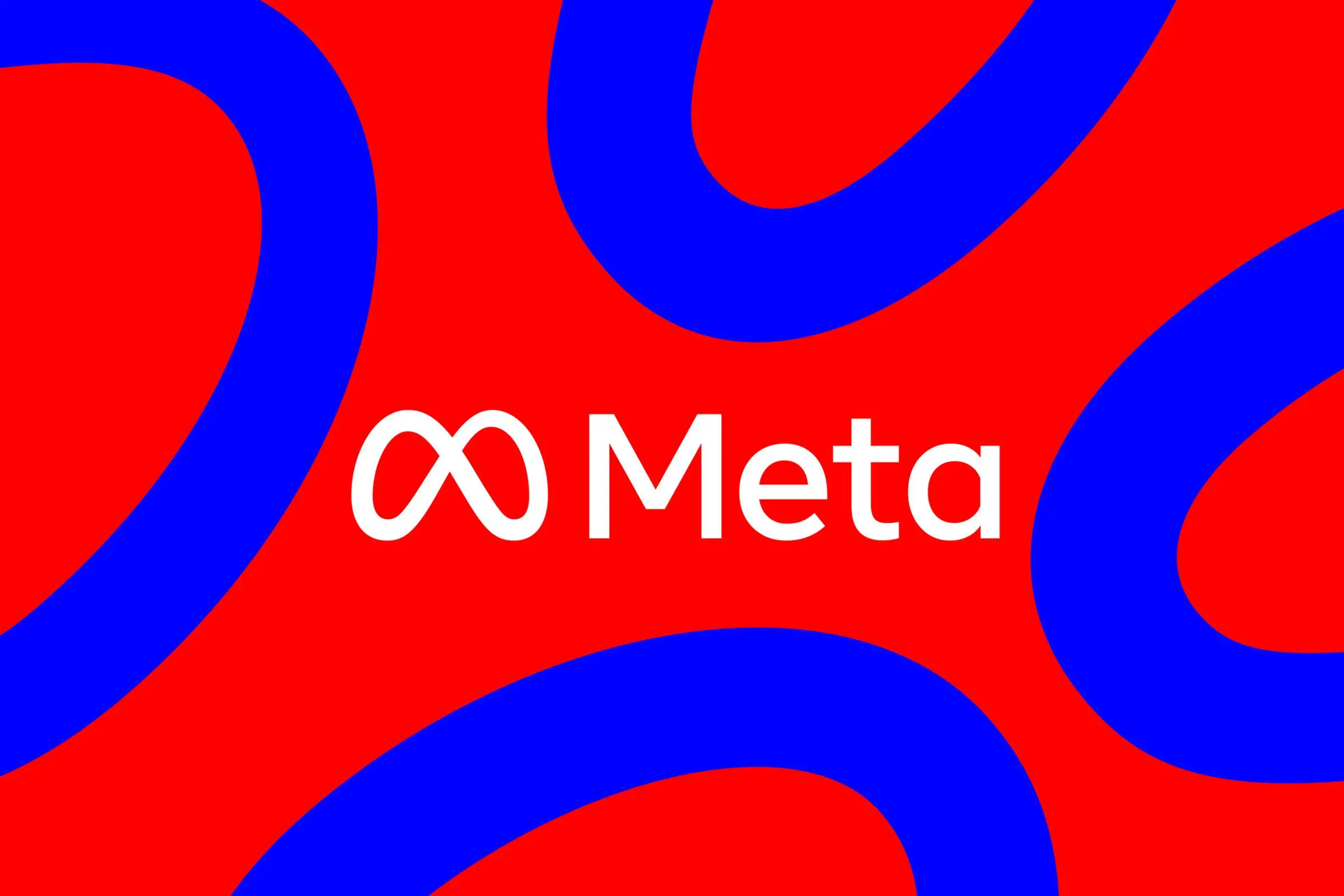
Meta is building its first custom chip specifically for running AI models, the company announced on Thursday. As Meta increases its AI efforts — CEO Mark Zuckerberg recently said the company sees “an opportunity to introduce AI agents to billions of people in ways that will be useful and meaningful” — the chip and other infrastructure plans revealed Thursday could be critical tools for Meta to compete with other tech giants also investing significant resources into AI.
Meta’s new MTIA chip, which stands for Meta Training and Inference Accelerator, is its “in-house, custom accelerator chip family targeting inference workloads,” Meta VP and head of infrastructure Santosh Janardhan wrote in a blog post. The chip apparently provides “greater compute power and efficiency” than CPUs and is “customized for our internal workloads.” With a combination of MTIA chips and GPUs, Janardhan said that Meta believes “we’ll deliver better performance, decreased latency, and greater efficiency for each workload.”